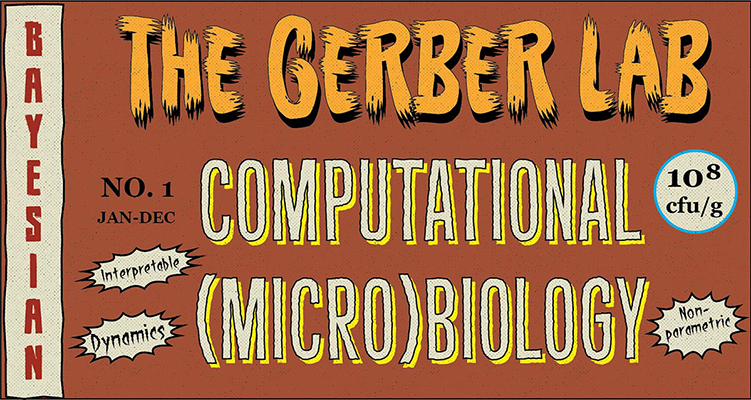
“Creating novel computational models and high-throughput experimental systems to understand the role of the microbiota in human diseases, and applying these findings to develop new diagnostic tests and therapeutic interventions to improve patient care.“
email: ggerber#bwh.harvard.edu, physical address: Hale Building 8002M, 60 Fenwood Road, Boston, MA 02115
Positions
- Research Scientist, Machine Learning and Computational Biology for MicrobiomeThe Microbiome AI/Deep Learning Lab in the Massachusetts Host-Microbiome Center and Division of Computational Pathology at Brigham and Women’s Hospital/Harvard Medical School is seeking a computational scientist with experience in machine learning. You will develop, deploy, and apply machine learning approaches, with a special emphasis on deep learning, to a variety of microbiology data sources.… Read more: Research Scientist, Machine Learning and Computational Biology for Microbiome
- Post Doctoral Fellow in Deep Learning for Microbiome Spatial OmicsThe Gerber Lab (http://gerber.bwh.harvard.edu) is a multidisciplinary group at Brigham and Women’s Hospital/Harvard Medical School that develops novel computational models and high-throughput experimental systems to understand the role of the microbiota in human diseases, and applies these findings to develop new diagnostic tests and therapies. A long-standing and continuing focus of the lab is on… Read more: Post Doctoral Fellow in Deep Learning for Microbiome Spatial Omics
- Graduate StudentsI am always excited to work with talented graduate students with interests relevant to my lab, which focuses on developing novel machine learning/computational biology/wet lab approaches to further understanding of the microbiome–the trillions of microbes living on and within us. This fascinating, complex and dynamic ecosystem is crucial for human health, and when disrupted may… Read more: Graduate Students
Lab News
- Dr. Gerber to speak at AAAS Annual meeting on Feb. 14, 2025 in BostonDr. Gerber will speak at the American Association for Advancement of Science Annual meeting, “Science Shaping Tomorrow” at the Hynes Convention Center in Boston.
- MMETHANE: interpretable AI for predicting host status from microbial composition and metabolomics dataWork led by Jen Dawkins – see our new manuscript. Metabolite production, consumption, and exchange are intimately involved with host health and disease, as well as being key drivers of host-microbiome interactions. Despite the increasing prevalence of datasets that jointly measure microbiome composition and metabolites, computational tools for linking these data to the status of… Read more: MMETHANE: interpretable AI for predicting host status from microbial composition and metabolomics data
- MCSPACE: inferring microbiome spatiotemporal dynamics from high-throughput co-localization dataWork led by Gary Uppal – see our new manuscript. Recent advances in high-throughput approaches for estimating co-localization of microbes, such as SAMPL-seq, allow characterization of the biogeography of the gut microbiome longitudinally and at unprecedented scale. However, these high-dimensional data are complex and have unique noise properties. To address these challenges, we developed MCSPACE,… Read more: MCSPACE: inferring microbiome spatiotemporal dynamics from high-throughput co-localization data
- “AI in microbiome research: Where have we been, where are we going?”Dr. Gerber lays out his perspective on how AI will impact microbiome research in this Cell Host & Microbe piece.
- Dr. Gerber to speak at the Nestle Nutrition Institute Workshop in Rio de Janeiro, Brazil on June 18, 2024Dr. Gerber will speak at the 101st Nestlé Nutrition Institute workshop – Nutrition, microbiome and health: latest findings and future research. The NNI and WNSC workshop 101 brought together renowned leading experts for a deep dive into the latest discoveries on the gut microbiome. Among the topics discussed were the ecological patterns occurring in early… Read more: Dr. Gerber to speak at the Nestle Nutrition Institute Workshop in Rio de Janeiro, Brazil on June 18, 2024