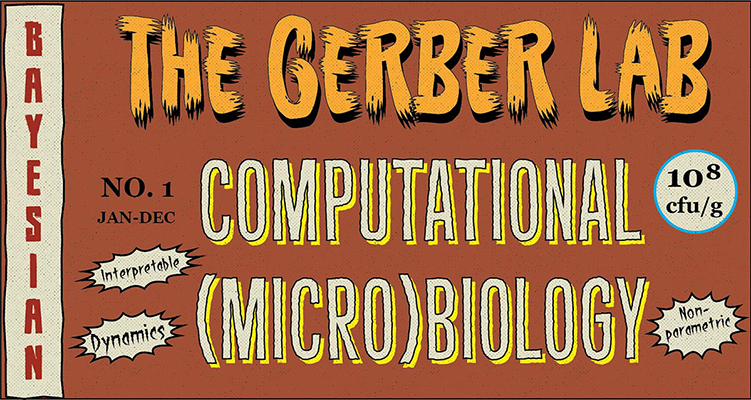
“Creating novel computational models and high-throughput experimental systems to understand the role of the microbiota in human diseases, and applying these findings to develop new diagnostic tests and therapeutic interventions to improve patient care.“
email: ggerber#bwh.harvard.edu, physical address: Hale Building 8002M, 60 Fenwood Road, Boston, MA 02115
Positions
- Post Doctoral Fellow in Deep Learning for Microbiome Spatial OmicsThe Gerber Lab (http://gerber.bwh.harvard.edu) is a multidisciplinary group at Brigham and Women’s Hospital/Harvard Medical School that develops novel computational models and high-throughput experimental systems to understand the role of the microbiota in human diseases, and applies these findings to develop new diagnostic tests and therapies. A long-standing and continuing focus of the lab is on… Read more: Post Doctoral Fellow in Deep Learning for Microbiome Spatial Omics
- Graduate StudentsI am always excited to work with talented graduate students with interests relevant to my lab, which focuses on developing novel machine learning/computational biology/wet lab approaches to further understanding of the microbiome–the trillions of microbes living on and within us. This fascinating, complex and dynamic ecosystem is crucial for human health, and when disrupted may… Read more: Graduate Students
Lab News
- Gerber Lab awarded $3.1 Million Five Year NIH-NIGMS R35 Grant “Probabilistic deep learning models and integrated biological experiments for analyzing dynamic and heterogeneous microbiomes”This work will leverage deep learning technologies to advance the microbiome field beyond finding associations in data, to accurately predicting the effects of perturbations on microbiota, elucidating mechanisms through which the microbiota affects the host, and improving bacteriotherapies to enable their success in the clinic. New deep learning models will be developed that address specific… Read more: Gerber Lab awarded $3.1 Million Five Year NIH-NIGMS R35 Grant “Probabilistic deep learning models and integrated biological experiments for analyzing dynamic and heterogeneous microbiomes”
- Christine Tataru, PhD, Data ScientistWelcome to the lab, Christine!
- Jiening Zhu, PhD, Postdoctoral FellowWelcome to the lab, Jiening!
- Two papers accepted at ICML WCB 2023Gerber GK, Bhattarai SK, Du M, Glickman MS, Bucci V. Discovery of Host-Microbiome Interactions Using Multi-Modal, Sparse, Time-Aware, Bayesian Network-Structured Neural Topic Models. International Conference on Machine Learning Workshop on Computational Biology, 2023. Uppal G, Urtecho G, Richardson M, Moody T, Wang HH, Gerber GK. MC-SPACE: Microbial communities from spatially associated counts engine. International Conference on Machine Learning… Read more: Two papers accepted at ICML WCB 2023
- “MDITRE: Scalable and Interpretable Machine Learning for Predicting Host Status from Temporal Microbiome Dynamics” is mSystems Editor’s PickLongitudinal microbiome data sets are being generated with increasing regularity, and there is broad recognition that these studies are critical for unlocking the mechanisms through which the microbiome impacts human health and disease. However, there is a dearth of computational tools for analyzing microbiome time-series data. To address this gap, we developed an open-source software… Read more: “MDITRE: Scalable and Interpretable Machine Learning for Predicting Host Status from Temporal Microbiome Dynamics” is mSystems Editor’s Pick